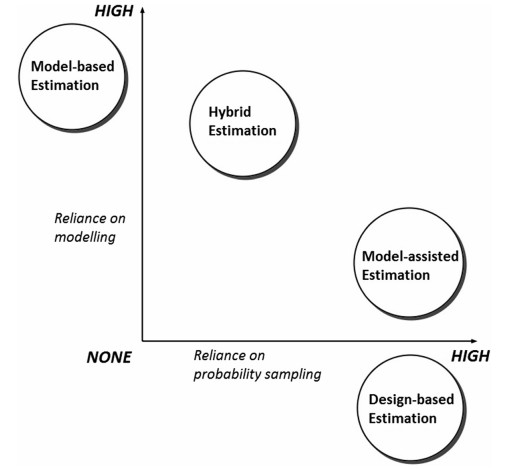
Citation: | Göran Ståhl, Svetlana Saarela, Sebastian Schnell, Sören Holm, Johannes Breidenbach, Sean P. Healey, Paul L. Patterson, Steen Magnussen, Erik Næsset, Ronald E. McRoberts, Timothy G. Gregoire. Use of models in large-area forest surveys: comparing model-assisted, model-based and hybrid estimation[J]. Forest Ecosystems, 2016, 3(1): 5-5. DOI: 10.1186/s40663-016-0064-9 |
Use of models in large-area surveys of forests is attracting increased interest. The reason is the improved availability of auxiliary data from various remote sensing platforms. Aerial photographs (e.g., Næsset 2002a, Bohlin et al. 2012) and optical satellite data (e.g., Reese et al. 2002) have been available and used operationally for many decades, while data from profiling (e.g., Nelson et al. 1984, Nelson et al. 1988) and scanning lasers (e.g., Næsset 1997) and radars (Solberg et al. 2010) have become available for practical applications more recently. Some of the new types of remotely sensed data, such as data from laser scanners, have already become widely applied in forest inventories (e.g., Næsset 2002b). A common application involves the development of models that are applied wall-to-wall over an area of interest (e.g., Næsset 2004), often for providing data for forest management. However, this type of data is increasingly applied also in connection with large-area forest surveys, such as national-level forest inventories (Tomppo et al. 2010, Asner et al. 2012).
Applications of models in large-area forest surveys often use the model-assisted estimation framework (Särndal et al. 1992) where a model is used to support the estimation following probability sampling within the context of design-based inference (Gregoire 1998). Importantly, an inadequately specified model will not make the estimators biased in this case, but only affect the variance of the estimators. Examples of large-area forest inventory applications include Andersen et al. (2011) who applied the technique in Alaska, Gregoire et al. (2011) and Gobakken et al. (2012), who applied it in Hedmark County, Norway, and Saarela et al. (2015a) who used it in Kuortane, Finland.
Some applications of models in large-area forest surveys involve model-based inference (Gregoire 1998), which to a larger extent than model-assisted estimation relies on model assumptions. In this case an inadequately specified model might make the estimators both biased and imprecise. On the other hand, with accurate models this mode of inference can be very efficient (e.g., Magnussen 2015). Examples of applications in forest inventory include McRoberts(2006, 2010), who used model-based inference for estimating forest area based on Landsat data in northern Minnesota, U.S.A., Ståhl et al. (2011) who used it for estimating biomass in Hedmark, Norway, using laser data, and Healey et al. (2012) who applied the technique in California, U.S.A., using data from the space-borne Geoscience Laser Altimeter System (GLAS).
Non-parametric modelling, applying methods such as the k-Nearest Neighbours (kNN) technique (Tomppo and Katila 1991, Tomppo et al. 2008), has a long tradition in forest inventories. These techniques typically have been applied for providing small-area estimates through combining field sample plots and various sources of remotely sensed data. However, the kNN technique has also been used in connection with model-assisted estimation (e.g., Baffetta et al. 2009, 2011, Magnussen and Tomppo 2015) and model-based inference (e.g., McRoberts et al. 2007).
The objective of this paper was to present, review and discuss how models are applied in the case of model-assisted and model-based estimation in large-area forest surveys, and to discuss advantages and disadvantages of the two estimation frameworks in this context. We also present, review and discuss a newly introduced estimation framework where probability sampling is applied for the selection of auxiliary data, upon which model-based inference is applied in a second phase. This framework in denoted hybrid inference, after Corona et al. (2014).
We restrict the study to large-area estimation. This is the case of national forest inventories and greenhouse gas inventories under the United Nations Framework Convention on Climate Change (e.g., Tomppo et al. 2010). Importantly, in this case there is no need to make assumptions about residual error terms linked to individual population elements, which is a core issue in model-based small-area estimation (e.g., Breidenbach and Astrup 2012, Breidenbach et al. 2015). The reason is that the residual error terms will have almost no influence on the results, as will be demonstrated below. However, we do not specify how large a "large area" must be, but use the term as a general concept.
Below, we present the basics of model-assisted, model-based, and hybrid inference (chapter 2). Subsequently we present a brief review of the application of these methods in forest surveys (chapter 3), and, finally, we discuss advantages and disadvantages of the different approaches and make conclusions (chapters 4 and 5).
In this chapter we summarize some basic concepts related to the use of models in large-area forest surveys. We restrict the scope to cases where models are applied for improving estimators (or predictors) once sample or wall-to-wall data have been collected. However, models may also be used in the design phase for improving the sample selection (e.g., Fattorini et al. 2009, Grafström et al. 2014), but such cases are not covered in this article.
This paper requires a basic understanding of the concepts design-based and model-based inference (e.g., Cassel et al. 1977, Särndal 1978, Gregoire 1998, McRoberts 2010).
Design-based inference typically assumes a finite population of elements to which one or more fixed target quantities are linked. The objective normally is to estimate some fixed population parameter, such as the total or the mean of these quantities (e.g., Gregoire and Valentine 2008). In order to estimate the fixed but unknown parameters a probability sample is selected from the population according to some appropriate sampling design, which assigns positive inclusion probabilities to each element. Mathematical formulas (estimators) are used for estimating the parameters based on the sample data. The estimates are random variables due to the random selection of samples, i.e., the estimators produce different values depending on which population elements are included in the sample.
The Horvitz-Thompson estimator can be applied to any probability sampling design with inclusion probabilities known at least for the sampled units (e.g., Särndal et al. 1992). Using this estimator, a population total, τ, is estimated as
ˆτ=∑i∈syiπi | (1) |
Here, yi is the variable of interest for the i:th sampled element, πi is the inclusion probability, and s is the sample.
The precision of an estimator is usually expressed through its variance, which is a fixed quantity given the population, the design, and the estimator. The variance usually can be estimated through a variance estimator, and confidence intervals can be computed as a means to provide decision makers with the range of values wherein the true population parameter is located with a defined probability.
In case of the Horvitz-Thompson estimator, a general formula for the variance is
var(ˆτ)=∑i∈U∑j∈U(πij−πiπj) yiπi yjπj | (2) |
In addition to the previously introduced notation, πij is the joint probability of inclusion for unit i and j. The step from the variance to a variance estimator and a confidence interval normally is straightforward (e.g., Gregoire and Valentine 2008).
Some key features of design-based inference are:
● The values that are linked to the population elements are fixed
● The population parameters about which we wish to infer information are also fixed
● Our estimators of the parameters are random because a probability sample is selected according to some sampling design, such as simple random sampling
● The probability of obtaining different samples can be deduced from the design and used for inference
The foundations of design-based inference were laid out by Neyman (1934) and it is the standard mode of inference in most statistical surveys, including sample-based national forest inventories (Tomppo et al. 2010) that are carried out in a large number of countries.
Models can be used to improve estimators under the design-based framework. An important category of such estimators are known as model-assisted estimators (Särndal et al. 1992). The general form of such estimators, for estimating a population total, is
ˆτma=∑i∈Uˆyi+∑i∈s(yi−ˆyi)πi | (3) |
where the first part of the estimator is a sum of model estimates of each element in the population; the second term is a Horvitz-Thompson estimator of the total of the deviations between observed values and values estimated by the model; the subscript 'ma' is used to point out that the estimator is model-assisted. Thus, the model-assisted estimator can be seen as composed of a first crude estimator which is refined through a correction term that makes it asymptotically unbiased when the model is external (in which case Eq. 3 is often referred to as a difference estimator), and approximately unbiased when the model is internal (in which case Eq. 3 is often referred to as a generalised regression estimator). In case the model is external the variance is
var(ˆτma)=∑i∈U∑j∈U(πij−πiπj) eiπi ejπj | (4) |
This is almost the same expression as the variance in Eq. (2), but the yi- terms have been replaced by ei = yi - ŷi. If an accurate model is used the latter terms should be much smaller than the former, and thus the variance of the model-assisted estimator should be much smaller than the variance of the ordinary Horvitz-Thompson estimator, although this is not immediately clear when comparing Eq. 2 and Eq. 4.
In contrast to design-based inference (including model-assisted estimators), a basic assumption underlying model-based inference is that the values that are linked to the elements in the population are realizations of random variables. As a consequence, target survey quantities such as population totals and means are also random variables. Thus, due to the different points of view underlying design-based and model-based inference some caution must be exercised when comparing results from the two inferential frameworks. For example, with model-based inference the random population total (or mean) may be predicted or (as in this study) the expected value of the population total may be estimated. For large population the difference between these two quantities, in relative terms, typically is minor although for small populations the relative difference may be substantial. However, just like design-based inference, model-based inference in many cases is a useful and straightforward approach for quantifying target features of a population (e.g., Chambers and Clark 2012). In forest inventories, examples of such cases are surveys of remote areas with poor road infrastructure and small-area estimation for forest management. In both cases the field sample sizes typically are small or acquired through non-probability sampling whereas remotely sensed data are available wall-to-wall.
A basic assumption of model-based inference is that the random values of the population elements follow some specific model, e.g., a model based on auxiliary data derived from remote sensing. Thus, in the standard case, auxiliary data are available for all population elements. A simple and fairly general example is the linear model, i.e., (in matrix form)
\boldsymbol{Y} = \bf{X}\boldsymbol{\beta } + \epsilon | (5) |
where Y is an N × 1 matrix of the target variable, X an N × p matrix of auxiliary data, β is a p × 1 matrix of model parameters, and ϵ an N × 1 matrix of random variables that follow some joint probability distribution; N is the population size; in a forest survey it might be the number of grid cells which tessellate the study area.
Our objective typically is to predict a random population quantity, e.g., the mean or the total, following the selection of a sample for estimating the model parameters. Regardless of how the sample is selected, the observations are realizations of random variables due to the model assumptions. Once the model parameters are estimated, we can use the estimated model, \widehat{\boldsymbol{Y}} = \boldsymbol{X}\widehat{\boldsymbol{\beta}} , for predicting the population quantities of interest based on the auxiliary data; in standard cases these are assumed available for all population elements. Introducing 1 as an N × 1 vector of "1"-entries, the random population total τ* = 1′Y = 1′Xβ + 1′ε may be predicted as
{\widehat{\tau}}^{*} = {\bf{1}}^{\bf{\prime}}\widehat{\boldsymbol{Y}} | (6) |
Note the distinction in nomenclature between estimating a fixed but unknown value (a population parameter) and predicting a random variable (e.g., Särndal 1978, Gregoire 1998). Note also that some authors (Chambers and Clark 2012) present the model-based predictor as a sum of two terms: the sum of the values of the sampled elements and the sum of the predictions for the non-sampled elements. The difference between such a predictor and Eq. (6) would, however, be very small in case a small sample is selected from a large population.
Turning to the mean square error of the predictor in Eq. (6) we need to acknowledge that uncertainty is introduced both by the estimation of the model parameters and by the random residual terms linked to each population element. Since the residuals may often be spatially auto-correlated estimating the mean square error of the Eq. (6) predictor may be very complicated.
However, an important feature of large-area surveys is that the relative difference between τ* and E (τ*) typically is very small (e.g., Chambers and Clark 2012, p. 16). The relative difference is 1′ε/(1′Xβ + 1′ε), which intuitively can be seen to tend to zero as N tends to infinity, since in the cases we focus on the Xiβ-terms are almost always positive and typically much larger (in absolute value) than the residual terms, which may be either negative or positive. Thus, instead of predicting τ*, in large-area estimation we can estimate E (τ*), which simplifies the model-based inference. The estimator will be identical to Eq. (6), i.e., \widehat{E\left({\tau}^{*}\right)} = {\bf{1}}^{\bf{\prime}}\widehat{\bf{Y}} , but it is now an estimator rather than a predictor. The variance (due to the model) of this estimator is simpler to derive, since it does not involve any residual terms; thus uncertainty in this case is introduced only through the model parameter estimation.
The variance of the estimator of E (τ*) is
var\left(\widehat{E\left[{\tau}^{*}\right]}\right)={\bf{1}}^{\bf{\prime}}\boldsymbol{Xcov}\left(\widehat{\boldsymbol{\beta}}\right){\boldsymbol{X}}^{\boldsymbol{\prime}}\bf{1} | (7) |
The matrix \boldsymbol{c}\boldsymbol{o}\boldsymbol{v}\left(\widehat{\boldsymbol{\beta}}\right) is the variance-covariance matrix of the model parameter estimates. A variance estimator is obtained by inserting the estimated covariance matrix in Eq. (7).
Thus, some key features of model-based inference are:
● The values linked to population elements are random variables
● Since the individual values are random variables so is the population total or mean that we wish to predict
● A model for the relationship between the target variable and one or more auxiliary variable(s) can adequately conform to the trend in Y.
● Auxiliary data are commonly available for all population elements
● After having selected a sample - that need not be random - for estimating the model parameters, we apply the fitted model for predicting the target population quantity or estimating the expected value of this quantity.
Auxiliary data may not be available prior to a forest survey and they may be very expensive to collect for all units in a population, as required for standard application of model-based inference. In such cases a probability sample of auxiliary data can be acquired, based on which the population total or mean of the auxiliary variable is estimated following design-based inference. A model can still be specified and applied regarding the relationship between the study variable and the auxiliary variables, and thus model-based inference can be applied once the auxiliary variable totals (or means) have been estimated through design-based inference.
Thus, design-based principles are applied in a first phase and model-based principles in a second phase. This approach was termed hybrid inference by Corona et al. (2014) and in the present paper we follow that terminology. In a previous study by Mandallaz (2013) it was called pseudo-synthetic estimation. In a study by Ståhl et al. (2011) it was simply called model-based inference, although later denoted model-dependent estimation by Gobakken et al. (2012). However, the term model-dependent estimation appears to have been first proposed by Hansen et al.(1978, 1983) to include all sampling strategies that depend on the correctness of a model; according to Hansen et al. (1978) "a model-dependent design consists of a sampling plan and estimators for which either the plan or the estimators, or both, are chosen because they have desirable properties under an assumed model, and for which the validity of inferences about the population depends on the degree to which the population conforms to the assumed model." Thus, standard model-based inference as well as hybrid inference, and other approaches, belong to Hansen's model-dependent category.
In the case of hybrid inference, expected values and variances are derived by considering both the design through which auxiliary data were collected and the model used for predicting values of population elements based on the auxiliary data. Thus, assuming we use a linear model, a general estimator of E (τ*) is given as
\widehat{E\left({\tau}^{*}\right)}={\displaystyle {\sum}_{i\in s}\frac{{\boldsymbol{X}}_{\boldsymbol{i}}\ \widehat{\boldsymbol{\beta}}}{\pi_i}={\boldsymbol{\pi}}^{\boldsymbol{\prime}}\boldsymbol{X}\widehat{\boldsymbol{\beta}}} | (8) |
where s is the sample of auxiliary data, πi is the probability of including population element i into the auxiliary data sample, π is an n-length column vector of (1/πi) - values, and X is an n × p matrix of sampled auxiliary data. The model parameters are estimated from a sample that is assumed to be independent from the sample of auxiliary data.
In deriving the variance of the estimator in Eq. (8), note that the part π′X of the estimator is a 1 × p matrix of design-unbiased estimators of population totals of auxiliary data, which we denote {\widehat{\tau}}_{\boldsymbol{x}} . This matrix is multiplied by the matrix of estimated model parameters, i.e., the result is a sum of estimated population totals of auxiliary variables times the corresponding model parameter estimate, such as {\widehat{\tau}}_{Xj} \cdot {\widehat{\beta}}_j . In each term the two components are independent, but the estimators of the auxiliary variable totals as well as the estimators of the parameters are typically correlated. Thus, the variance (due to the sample and the model) is
\begin{array}{l}var\left(\widehat{E\left[{\tau}^{*}\right]}\right)=var\left({\widehat{\boldsymbol{\tau}}}_{\boldsymbol{x}}\widehat{\boldsymbol{\beta}}\right) = {\boldsymbol{\beta}}^{\boldsymbol{\prime}}\boldsymbol{c}\boldsymbol{o}\boldsymbol{v}\left({\widehat{\boldsymbol{\tau}}}_{\boldsymbol{x}}\right)\boldsymbol{\beta} \\+ {\boldsymbol{\tau}}_{\boldsymbol{x}}\boldsymbol{c}\boldsymbol{o}\boldsymbol{v}\left(\widehat{\boldsymbol{\beta}}\right){\boldsymbol{\tau}}_{\boldsymbol{x}}^{\boldsymbol{\prime}} + Tr\left(\boldsymbol{c}\boldsymbol{o}\boldsymbol{v}\left({\widehat{\boldsymbol{\tau}}}_{\boldsymbol{x}}\right)\boldsymbol{c}\boldsymbol{o}\boldsymbol{v}\left(\widehat{\boldsymbol{\beta}}\right)\right)\\ {}\end{array} | (9) |
where \boldsymbol{c}\boldsymbol{o}\boldsymbol{v}\left({\widehat{\tau}}_{\boldsymbol{x}}\right) is the covariance matrix of the estimators of the auxiliary variable totals and \boldsymbol{c}\boldsymbol{o}\boldsymbol{v}\left(\widehat{\boldsymbol{\beta}}\right) is the covariance matrix of the model parameter estimators. The Tr-operator is the trace, i.e., the sum of the diagonal entries in the matrix. The diagonal entries in \boldsymbol{c}\boldsymbol{o}\boldsymbol{v}\left({\widehat{\tau}}_{\boldsymbol{x}}\right) are of the kind presented in Eq. (2). The off-diagonal entries are computed in a similar fashion (Särndal et al. 1992). The covariance matrix of the model parameter estimators normally, under ordinary least squares regression assumptions, is derived as σ2(X′X)- 1 where σ2 is the residual variance, given the regression model. In case of heteroskedastic residual variance, alternative estimators can be applied (e.g., Saarela et al. 2015b). We do not offer a proof of Eq. (9), but readers familiar with the variance of a product of two independent random variables (i.e., var(WZ) = E(W)2var(Z) + E(Z)2var(W) + var(W)var(Z)) can identify the similarity with Eq. (9).
Although it seems likely that hybrid type estimators have been applied outside forest inventories, we have not yet found any description of them in non-forest publications.
In Fig. 1 an overview of the "positions" of standard design-based estimation (without using models), model-assisted estimation, hybrid estimation, and model-based estimation is shown with regard to how much these estimation techniques rely on (ⅰ) the correctness of the model and (ⅱ) the use of probability sampling.
From the methods section it is clear that models can be used in several ways for improving the estimation of target quantities in large-area forests surveys. Our review is separated into the following cases:
● Use of models in the context of design-based inference through model-assisted estimation
● Use of models in the context of model-based inference through model-based estimation
● Use of models in the context of hybrid inference
Formal model-assisted estimators appear to be fairly recently introduced to large-area forest surveys, although standard regression estimators (i.e., a simple kind of model-assisted estimators) have been applied in forest surveys for a long time. An important example of the latter kind is the Swiss national forest inventory (Köhl and Brassel 2001) where air photo interpretation has been combined with field surveys for a long time and the Italian national forest inventory, where a three-phase sampling approach is applied (Fattorini et al. 2006).
An early model-assisted study was conducted by Breidt et al. (2005), who used spline models in estimating population totals in a simulation study linked to surveys of forest health. Model-assisted estimation was found to perform well in the context of a two-phase survey with multiple auxiliary variables.
Opsomer et al. (2007) used model-assisted estimation in a two-phase systematic sampling design, applying generalized additive models linking ground measurements with auxiliary information from remote sensing. The study was an extension of the study by Breidt and Opsomer (2000), where univariate models and a single-phase sampling strategy were applied.
In Boudreau et al. (2008), model-assisted estimation was used for estimating biomass in Quebec, Canada, based on data from a laser profiler, GLAS satellite data, and land cover maps based on data from Landsat-7 ETM+. The study demonstrated that GLAS data could improve large-scale monitoring of aboveground biomass at large spatial scales; however, the presented estimators were not denoted "model-assisted". Nelson et al. (2009) built upon the study by Boudreau et al. (2008) and introduced some new, partly model-based, estimation techniques. Andersen et al. (2009) presented a study based on model-assisted estimation where the biomass of western Kenai, Alaska, was estimated based on samples of field and laser scanner data.
In Gregoire et al. (2011) model-assisted estimation was used for estimating aboveground biomass in Hedmark County, Norway, using sample data from laser profilers and scanners. The study triggered the start of a series of studies where the model-assisted theory, developed by Särndal et al. (1992), was applied for large-scale forest surveys based on samples of laser scanner data. Næsset et al. (2011) applied and compared two sources of auxiliary information, laser scanner data and interferometric synthetic aperture radar data for model-assisted estimation of biomass over a large boreal forest area in the Aurskog-Høland municipality in Norway and quantified to what extent the two types of auxiliary data improved the estimated precision. Gobakken et al. (2012) compared the performance of model-assisted estimation with model-based prediction of aboveground biomass in Hedmark County, Norway using data from airborne laser scanning as auxiliary data. The two approaches were found to yield similar results. Nelson et al. (2012) conducted a similar study over the same area using data from a profiling rather than scanning airborne laser, while Næsset et al. (2013b) evaluated the precision of the two-stage model-assisted estimation conducted by Gobakken et al. (2012). The authors noted the sensitivity of variance estimators to unequal sample strip length and systematically selected strips. The latter issue was further pursued by Ene et al. (2012), who showed that the variance was often severely overestimated when estimators assuming simple random sampling were applied in this context. Similar results were reported by Magnussen et al. (2014).
Strunk et al.(2012a, 2012b) investigated different aspects of model-assisted estimation. For example, the authors found that the laser pulse density had almost no effect on the precision of model-assisted estimators of core parameters, such as basal area, volume, and biomass.
Saarela et al. (2015a) proposed to use probability-proportional-to-size sampling of laser scanning strips in a two-phase model-assisted sampling study where the total growing stock volume was estimated in a boreal forest area in Kuortane, Finland. It was also found that full cover of Landsat auxiliary information improved the precision of estimators compared to using only sampled LiDAR strip data.
Massey et al. (2014) evaluated the performance of the model-assisted estimation technique in connection with the Swiss national forest inventory. The authors also addressed several methodological issues and, among other things, evaluated the performance of non-parametric methods in connection with model-assisted estimation and the close connection between difference estimators and regression estimators.
As some of the first laser scanning campaigns carried out for inventory purposes at the turn of the millennium have been repeated in recent years, change estimation assisted by laser data have become an important research area. Bollandsås et al. (2013), Næsset et al.(2013a, 2015), Skowronski et al. (2014), McRoberts et al. (2015), and Magnussen et al. (2015) analysed different approaches to modelling of change in biomass, such as separate modelling of biomass at each point in time and then estimate the difference, direct modelling of change with different predictor variables, such as the variables at each time point or their differences, and longitudinal models. These modelling techniques have been combined with different design-based and model-based estimators to produce change estimates and confidence intervals. Sannier et al. (2014) investigated change estimation based on a series of maps, which provided the auxiliary data for model-assisted difference estimation. A comprehensive review and discussion of change estimation can be found in McRoberts et al.(2014, 2015). Melville et al. (2015) evaluated three model-based and three design-based methods for assessing the number of stems using airborne laser scanning data. The authors reported that among the design-based estimators, the most precise estimates were achieved through stratification.
Stephens et al. (2012) applied double sampling regression estimators in the design-based framework for estimating carbon stocks in New Zealand forests using laser data as auxiliary information.
Chirici et al. (2016) compared the performance of two types of airborne LiDAR-based metrics in estimating total aboveground biomass through model-assisted estimators. The study area was located in Molise Region in central Italy. Corona et al. (2015) dealt with the use of map data as auxiliary information in a similar context.
McRoberts(2006, 2010) applied model-based inference for estimating forest area using Landsat data as auxiliary information and field plots data. The studies were performed in northern Minnesota, U.S.A. In the studies the expected value of the total forest area was estimated, as a means to reduce the complexity of the variance estimators.
A large number of studies have applied model-based prediction for mapping forest attributes across large areas using remotely sensed auxiliary information. Baccini et al. (2008) used moderate resolution imaging spectro-radiometer (MODIS) and GLAS for mapping aboveground biomass across tropical Africa. Armston et al. (2009) used Landsat-5 TM and Landsat-7 ETM+ sensors for prediction foliage projective cover across a large area in Queensland, Australia. Asner et al. (2010) applied model-based prediction for mapping the aboveground carbon stocks using satellite imaging, airborne LiDAR and field plots over 4.3 million ha of Peruvian Amazon. Helmer et al. (2010) used time series from 24 Landsat TM/ETM+ and Advance Land Imager (ALI) scenes for mapping forest attributes on the island of Eleuthera. These are only examples of a very large number of studies where wall-to-wall remotely sensed data have been applied for mapping and monitoring forest resources. However, a majority of these studies do not apply a formal model-based inferential framework. For example, in case the uncertainty of estimators is addressed, usually the strict model-based inference approach [Eq. (7)] is not applied but instead some other, often ad-hoc, method that does not correctly reflect the uncertainty of the estimator or predictor involved.
Saarela et al. (2015b) evaluated the effects of model form and sample size on the precision of model-based estimators in the study area Kuortane, Finland, and identified minor to moderate differences in results when different model forms were applied. In a simulation study, Magnussen (2015) demonstrated the usefulness of model-based inference for forest surveys and argued that this approach has several advantages over traditional design-based sampling. McRoberts et al.(2014a, b) assessed the effects of uncertainty in model predictions of individual tree volume model predictions on large-area volume estimates in the survey framework of hybrid inference.
As previously mentioned, Corona et al. (2014) proposed to use the term hybrid inference for the case where a probability sample of auxiliary data may be selected, on which model-based inference is applied; the study by Corona et al. mainly dealt with small-area estimation issues. Ståhl et al. (2011), Gobakken et al. (2012), Nelson et al. (2012) and Magnussen et al. (2014) used hybrid inference for estimating the forest resources in Hedmark county, Norway, based on combinations of laser scanner data, laser profiler data, and field data. In the study by Magnussen et al. two populations were simulated using the data. Healey et al. (2012) applied the technique in California, using GLAS data. In a study of boreal forests in Canada, Margolis et al. (2015) likewise used GLAS data, in combination with airborne laser data, to estimate aboveground biomass.
Geographical mismatches between remotely sensed data and field measurements may considerably affect the precision of estimators in large-area surveys. The effects of such errors in model-based and model-assisted estimation were evaluated by Saarela et al. (2016).
The findings from the brief literature review are summarized in Fig. 2.
The review revealed that use of models in large-scale forest inventories is widespread, although statistically strict applications of model-assisted estimators, model-based inference, or hybrid inference are rather limited. While the model-assisted estimation framework is attracting large interest, model-based inference and hybrid inference are not applied as much. A large number of studies apply approaches that could be classified as model-based inference, although they do not pursue any strict uncertainty analyses. In this context there is room for substantial improvement regarding how mean square errors or variances are estimated.
An advantage of model-assisted estimation, as compared to model-based and hybrid inference, is that the unbiasedness of estimators of totals and means do not rely on the correctness of the model; the model is only applied for enhancing a design-based estimator (Särndal et al. 1992). Whereas there is a theoretical chance that a model-assisted estimator is worse (in terms of variance) than a strictly design-based estimator if the model is extremely poor, a well specified model might substantially increase the precision of the model-assisted estimator compared to the strictly design-based estimator. This was shown by, e.g., Ene et al. (2012) and Saarela et al. (2015a).
If well specified models are available model-based inference is definitely a competitive alternative to design-based inference through model-assisted estimation (McRoberts et al. 2014a, b, Magnussen 2015). It has advantages since it does not rely on a probability sample from the target area. Such samples may sometimes not be feasible due to poor infrastructure conditions, restricted access to private land, or the presence of areas that are for some reason dangerous to visit in the field. Further, in case a probability sample has been selected, based upon which models are developed and applied, model-based inference and model-assisted estimation usually lead to similar total estimates. In case the condition {\displaystyle {\sum}_{i\in s}^n\frac{\left({y}_i-{\widehat{y}}_i\right)}{\pi_i}=0} holds the estimated values will be identical. However, Saarela et al. (2016) showed that the model-based variance estimators are less prone to problems with geolocation mismatches between field plots and remotely sensed auxiliary data.
Hybrid inference is a straightforward approach in cases where auxiliary data are not available wall-to-wall and such data are expensive to acquire. In such cases a sample of auxiliary data can be selected, upon which the auxiliary variable totals and means can be estimated and used together with model predictions that link the auxiliary variables with the target variable. The approach so far appears to have been applied only in a limited number of forest inventories, although implicitly it has been used for a long time in forest inventories where models (such as volume, biomass and growth models) have been applied based on data from forest plots (Ståhl et al. 2014).
Overall, the use of models relies on auxiliary data that are correlated with or otherwise related with the target variable. Considering the variables normally included in national forest inventories (Tomppo et al. 2010) it is likely that a large number of variables would be very difficult to model in terms of remotely sensed data. This might be the case for forest floor vegetation, soil properties, and several types of forest damage. Modelling approaches linked to such variables would probably not improve the precision of estimators. Thus, a large number of variables, such as site index, forest floor vegetation, soil type, etc., are likely to require probability field samples.
We conclude by noting that all three approaches studied: model-assisted estimation, model-based inference, and hybrid inference, have advantages and disadvantages when applied in large-area forest surveys. A main advantage of model-assisted estimation is that unbiasedness of estimators does not rely on the suitability of the model, but the model only helps to improve the precision of an estimator known to be (approximately) unbiased. Model-based and hybrid inference rely on the suitability of the model, but may have several advantages under conditions where access to field plots is difficult or expensive. All three approaches rely on the possibility to develop accurate models, which is possible for several important forest variables (such as biomass), but not for all variables that are included in a normal national forest inventory.
The authors declare that they have no competing interests.
GS: Initiative and major contribution to writing and review. SvS: Major contribution to writing and review. SeS, SH, JB, SPH, PLP, SM, EN, REM, TGG: Contribution to review and suggestions for improvement to preliminary versions of the manuscript. All authors read and approved the final manuscript.
[1] | Yan Zheng, Zhengyang Hou, Göran Ståhl, Ronald E. McRoberts, Weisheng Zeng, Erik Næsset, Terje Gobakken, Bo Li, Qing Xu. Nexus of certain model-based estimators in remote sensing forest inventory[J]. Forest Ecosystems, 2024, 11(1): 100245. DOI: 10.1016/j.fecs.2024.100245 |
[2] | Göran Ståhl, Terje Gobakken, Svetlana Saarela, Henrik J. Persson, Magnus Ekström, Sean P. Healey, Zhiqiang Yang, Johan Holmgren, Eva Lindberg, Kenneth Nyström, Emanuele Papucci, Patrik Ulvdal, Hans Ole Ørka, Erik Næsset, Zhengyang Hou, Håkan Olsson, Ronald E. McRoberts. Why ecosystem characteristics predicted from remotely sensed data are unbiased and biased at the same time – and how this affects applications[J]. Forest Ecosystems, 2024, 11(1): 100164. DOI: 10.1016/j.fecs.2023.100164 |
[3] | Zhengyang Hou, Ronald E. McRoberts, Chunyu Zhang, Göran Ståhl, Xiuhai Zhao, Xuejun Wang, Bo Li, Qing Xu. Cross-classes domain inference with network sampling for natural resource inventory[J]. Forest Ecosystems, 2022, 9(1): 100029. DOI: 10.1016/j.fecs.2022.100029 |
[4] | Matieu Henry, Zaheer Iqbal, Kristofer Johnson, Mariam Akhter, Liam Costello, Charles Scott, Rashed Jalal, Md. Akhter Hossain, Nikhil Chakma, Olaf Kuegler, Hossain Mahmood, Rajib Mahamud, Mohammad Raqibul Hasan Siddique, Khaled Misbahuzzaman, Mohammad Main Uddin, Mohammed Al Amin, Farid Uddin Ahmed, Gael Sola, Md. Baktiar Siddiqui, Luca Birigazzi, Mahmudur Rahman, Ilias Animon, Saimunnahar Ritu, Laskar Muqsudur Rahman, Aminul Islam, Heather Hayden, Frida Sidik, Mondal Falgoonee Kumar, Rakibul Hassan Mukul, Hossain Nishad, Ariful Hoque Belal, Asif Reza Anik, Abdul Khaleque, Md. Shaheduzzaman, Syed Shahadat Hossain, Tariq Aziz, Md. Tauhidor Rahaman, Ruhul Mohaiman, Patrick Meyer, Purnata Chakma, A.Z.M. Manzoor Rashid, Sourav Das, Shrabanti Hira, Mohammed Jashimuddin, Mohammad Mahfuzur Rahman, Karl Wurster, Sarder Nasir Uddin, Abul Kalam Azad, S.M. Zahirul Islam, Laurent Saint-André-Show. A multi-purpose National Forest Inventory in Bangladesh: design, operationalisation and key results[J]. Forest Ecosystems, 2021, 8(1): 12-12. DOI: 10.1186/s40663-021-00284-1 |
[5] | Johannes Schumacher, Marius Hauglin, Rasmus Astrup, Johannes Breidenbach. Mapping forest age using National Forest Inventory, airborne laser scanning, and Sentinel-2 data[J]. Forest Ecosystems, 2020, 7(1): 60-60. DOI: 10.1186/s40663-020-00274-9 |
[6] | Minna Räty, Mikko Kuronen, Mari Myllymäki, Annika Kangas, Kai Mäkisara, Juha Heikkinen. Comparison of the local pivotal method and systematic sampling for national forest inventories[J]. Forest Ecosystems, 2020, 7(1): 54-54. DOI: 10.1186/s40663-020-00266-9 |
[7] | Piermaria Corona, Lorenzo Fattorini, Maria Chiara Pagliarella. Sampling strategies for estimating forest cover from remote sensing-based two-stage inventories[J]. Forest Ecosystems, 2015, 2(1): 18-18. DOI: 10.1186/s40663-015-0042-7 |
[8] | Krishna P Poudel, Hailemariam Temesgen, Andrew N Gray. Evaluation of sampling strategies to estimate crown biomass[J]. Forest Ecosystems, 2015, 2(1): 1-1. DOI: 10.1186/s40663-014-0025-0 |
[9] | YANG Xiao-lin, ZHANG Xi-ming, LI Yi-ling, LI Shao-cai, SUN Hai-long. Analysis of root fractal characteristics in remote areas of the Taklimakan desert, China[J]. Forest Ecosystems, 2008, 10(2): 67-73. DOI: 10.1007/s11632-008-0024-8 |
[10] | WANG Lei, HUANG Hua-guo, ZHANG Xiao-li, LUO You-qing, SHI Juan. Monitoring of Pinus massoniana spatial pattern changes based on RS and GIS techniques[J]. Forest Ecosystems, 2008, 10(1): 32-35. DOI: 10.1007/s11632-008-0011-0 |
1. | Steven K. Filippelli, Karen Schleeweis, Mark D. Nelson, et al. Testing temporal transferability of remote sensing models for large area monitoring. Science of Remote Sensing, 2024, 9: 100119. DOI:10.1016/j.srs.2024.100119 |
2. | Ronald E. McRoberts, Erik Næsset, Juha Heikkinen, et al. Two-stage, model-assisted estimation using remotely sensed auxiliary data. Remote Sensing of Environment, 2024, 307: 114125. DOI:10.1016/j.rse.2024.114125 |
3. | Rajesh Malla, Prem Raj Neupane, Michael Köhl. Assessment of above ground biomass and soil organic carbon in the forests of Nepal under climate change scenario. Frontiers in Forests and Global Change, 2023, 6 DOI:10.3389/ffgc.2023.1209232 |
4. | Ronald E. McRoberts, Erik Næsset, Zhengyang Hou, et al. How many bootstrap replications are necessary for estimating remote sensing-assisted, model-based standard errors?. Remote Sensing of Environment, 2023, 288: 113455. DOI:10.1016/j.rse.2023.113455 |
5. | Qing Xu, Bo Li, Ronald E. McRoberts, et al. Harnessing data assimilation and spatial autocorrelation for forest inventory. Remote Sensing of Environment, 2023, 288: 113488. DOI:10.1016/j.rse.2023.113488 |
6. | Yue Wan, Jingxiong Zhang, Wangle Zhang, et al. Characterizing Uncertainty and Enhancing Utility in Remotely Sensed Land Cover Using Error Matrices Localized in Canonical Correspondence Analysis Ordination Space. Remote Sensing, 2023, 15(5): 1367. DOI:10.3390/rs15051367 |
7. | K C Cushman, John Armston, Ralph Dubayah, et al. Impact of leaf phenology on estimates of aboveground biomass density in a deciduous broadleaf forest from simulated GEDI lidar. Environmental Research Letters, 2023, 18(6): 065009. DOI:10.1088/1748-9326/acd2ec |
8. | Fangting Chen, Zhengyang Hou, Svetlana Saarela, et al. Leveraging remotely sensed non-wall-to-wall data for wall-to-wall upscaling in forest inventory. International Journal of Applied Earth Observation and Geoinformation, 2023, 119: 103314. DOI:10.1016/j.jag.2023.103314 |
9. | Eric L Bullock, Sean P Healey, Zhiqiang Yang, et al. Estimating aboveground biomass density using hybrid statistical inference with GEDI lidar data and Paraguay’s national forest inventory. Environmental Research Letters, 2023, 18(8): 085001. DOI:10.1088/1748-9326/acdf03 |
10. | Svetlana Saarela, Petri Varvia, Lauri Korhonen, et al. Three-phase hierarchical model-based and hybrid inference. MethodsX, 2023, 11: 102321. DOI:10.1016/j.mex.2023.102321 |
11. | Zhengyang Hou, Keyan Yuan, Göran Ståhl, et al. Conjugating remotely sensed data assimilation and model-assisted estimation for efficient multivariate forest inventory. Remote Sensing of Environment, 2023, 299: 113854. DOI:10.1016/j.rse.2023.113854 |
12. | James R. Kellner, John Armston, Laura Duncanson. Algorithm Theoretical Basis Document for GEDI Footprint Aboveground Biomass Density. Earth and Space Science, 2023, 10(4) DOI:10.1029/2022EA002516 |
13. | David M. Bell, Barry T. Wilson, Charles E. Werstak, et al. Examining k-Nearest Neighbor Small Area Estimation Across Scales Using National Forest Inventory Data. Frontiers in Forests and Global Change, 2022, 5 DOI:10.3389/ffgc.2022.763422 |
14. | J.P. Renaud, A. Sagar, P. Barbillon, et al. Characterizing the calibration domain of remote sensing models using convex hulls. International Journal of Applied Earth Observation and Geoinformation, 2022, 112: 102939. DOI:10.1016/j.jag.2022.102939 |
15. | Natalia Málaga, Sytze de Bruin, Ronald E. McRoberts, et al. Precision of subnational forest AGB estimates within the Peruvian Amazonia using a global biomass map. International Journal of Applied Earth Observation and Geoinformation, 2022, 115: 103102. DOI:10.1016/j.jag.2022.103102 |
16. | Garret T. Dettmann, Philip J. Radtke, John W. Coulston, et al. Review and Synthesis of Estimation Strategies to Meet Small Area Needs in Forest Inventory. Frontiers in Forests and Global Change, 2022, 5 DOI:10.3389/ffgc.2022.813569 |
17. | Todd A. Schroeder, Shingo Obata, Monica Papeş, et al. Evaluating Statewide NAIP Photogrammetric Point Clouds for Operational Improvement of National Forest Inventory Estimates in Mixed Hardwood Forests of the Southeastern U.S.. Remote Sensing, 2022, 14(17): 4386. DOI:10.3390/rs14174386 |
18. | Alexander Robitzsch, Oliver Lüdtke. Some thoughts on analytical choices in the scaling model for test scores in international large-scale assessment studies. Measurement Instruments for the Social Sciences, 2022, 4(1) DOI:10.1186/s42409-022-00039-w |
19. | Hans Ole Ørka, Marie-Claude Jutras-Perreault, Erik Næsset, et al. A framework for a forest ecological base map – An example from Norway. Ecological Indicators, 2022, 136: 108636. DOI:10.1016/j.ecolind.2022.108636 |
20. | David S. L. Ramsey, David M. Forsyth, Mike Perry, et al. Using helicopter counts to estimate the abundance of Himalayan tahr in New Zealand's Southern Alps. The Journal of Wildlife Management, 2022, 86(6) DOI:10.1002/jwmg.22252 |
21. | Diogo N. Cosenza, Petteri Packalen, Matti Maltamo, et al. Effects of numbers of observations and predictors for various model types on the performance of forest inventory with airborne laser scanning. Canadian Journal of Forest Research, 2022, 52(3): 385. DOI:10.1139/cjfr-2021-0192 |
22. | Henrik J. Persson, Kenneth Olofsson, Johan Holmgren. Two-phase forest inventory using very-high-resolution laser scanning. Remote Sensing of Environment, 2022, 271: 112909. DOI:10.1016/j.rse.2022.112909 |
23. | Ronald E. McRoberts, Erik Næsset, Juha Heikkinen, et al. On the model-assisted regression estimators using remotely sensed auxiliary data. Remote Sensing of Environment, 2022, 281: 113168. DOI:10.1016/j.rse.2022.113168 |
24. | Yifan Yu, Sassan Saatchi, Grant M Domke, et al. Making the US national forest inventory spatially contiguous and temporally consistent. Environmental Research Letters, 2022, 17(6): 065002. DOI:10.1088/1748-9326/ac6b47 |
25. | James A. Westfall, John W. Coulston, Gretchen G. Moisen, et al. Sampling and Estimation Documentation for the Enhanced Forest Inventory and Analysis Program. Sampling and Estimation Documentation for the Enhanced Forest Inventory and Analysis Program, 2022. DOI:10.2737/NRS-GTR-207 |
26. | Ankit Sagar, Cédric Vega, Olivier Bouriaud, et al. Multisource forest inventories: A model-based approach using k-NN to reconcile forest attributes statistics and map products. ISPRS Journal of Photogrammetry and Remote Sensing, 2022, 192: 175. DOI:10.1016/j.isprsjprs.2022.08.016 |
27. | Henrik Jan Persson, Magnus Ekström, Göran Ståhl. Quantify and account for field reference errors in forest remote sensing studies. Remote Sensing of Environment, 2022, 283: 113302. DOI:10.1016/j.rse.2022.113302 |
28. | Ioan Dutcă, Ronald E. McRoberts, Erik Næsset, et al. Accommodating heteroscedasticity in allometric biomass models. Forest Ecology and Management, 2022, 505: 119865. DOI:10.1016/j.foreco.2021.119865 |
29. | Ralph Dubayah, John Armston, Sean P Healey, et al. GEDI launches a new era of biomass inference from space. Environmental Research Letters, 2022, 17(9): 095001. DOI:10.1088/1748-9326/ac8694 |
30. | Adam P. Kubiak, Paweł Kawalec. Prior Information in Frequentist Research Designs: The Case of Neyman’s Sampling Theory. Journal for General Philosophy of Science, 2022, 53(4): 381. DOI:10.1007/s10838-022-09600-x |
31. | Juan Guerra-Hernández, Brigite Botequim, Sandra Bujan, et al. Interpreting the uncertainty of model-based and design-based estimation in downscaling estimates from NFI data: a case-study in Extremadura (Spain). GIScience & Remote Sensing, 2022, 59(1): 686. DOI:10.1080/15481603.2022.2051383 |
32. | Ronald E. McRoberts, Erik Næsset, Sassan Saatchi, et al. Statistically rigorous, model-based inferences from maps. Remote Sensing of Environment, 2022, 279: 113028. DOI:10.1016/j.rse.2022.113028 |
33. | Olek C. Wojcik, Samuel D. Olson, Paul-Hieu V. Nguyen, et al. GREGORY: A Modified Generalized Regression Estimator Approach to Estimating Forest Attributes in the Interior Western US. Frontiers in Forests and Global Change, 2022, 4 DOI:10.3389/ffgc.2021.763414 |
34. | Mathieu Fortin, Jean-François Lavoie. Reconciling individual-based forest growth models with landscape-level studies through a meta-modelling approach. Canadian Journal of Forest Research, 2022, 52(8): 1140. DOI:10.1139/cjfr-2022-0002 |
35. | Erik Næsset, Terje Gobakken, Marie-Claude Jutras-Perreault, et al. Comparing 3D Point Cloud Data from Laser Scanning and Digital Aerial Photogrammetry for Height Estimation of Small Trees and Other Vegetation in a Boreal–Alpine Ecotone. Remote Sensing, 2021, 13(13): 2469. DOI:10.3390/rs13132469 |
36. | Cédric Vega, Jean-Pierre Renaud, Ankit Sagar, et al. A new small area estimation algorithm to balance between statistical precision and scale. International Journal of Applied Earth Observation and Geoinformation, 2021, 97: 102303. DOI:10.1016/j.jag.2021.102303 |
37. | S. Puliti, J. Breidenbach, J. Schumacher, et al. Above-ground biomass change estimation using national forest inventory data with Sentinel-2 and Landsat. Remote Sensing of Environment, 2021, 265: 112644. DOI:10.1016/j.rse.2021.112644 |
38. | Efraín Velasco-Bautista, Martin Enrique Romero-Sanchez, David Meza-Juárez, et al. Assessment of the Effect of Six Methods of Analysis and Different Sample Sizes for Biomass Estimation in Grasslands of the State of Puebla, Mexico. Land, 2021, 10(11): 1194. DOI:10.3390/land10111194 |
39. | Lihou Qin, Shengwang Meng, Guang Zhou, et al. Uncertainties in above ground tree biomass estimation. Journal of Forestry Research, 2021, 32(5): 1989. DOI:10.1007/s11676-020-01243-2 |
40. | Magnus Ekström, Mats Nilsson. A Comparison of Model-Assisted Estimators, With and Without Data-Driven Transformations of Auxiliary Variables, With Application to Forest Inventory. Frontiers in Forests and Global Change, 2021, 4 DOI:10.3389/ffgc.2021.764495 |
41. | George C. Gaines, David L. R. Affleck. Small Area Estimation of Postfire Tree Density Using Continuous Forest Inventory Data. Frontiers in Forests and Global Change, 2021, 4 DOI:10.3389/ffgc.2021.761509 |
42. | Richard W. Guldin. A Systematic Review of Small Domain Estimation Research in Forestry During the Twenty-First Century From Outside the United States. Frontiers in Forests and Global Change, 2021, 4 DOI:10.3389/ffgc.2021.695929 |
43. | Máira Beatriz Teixeira da Costa, Carlos Alberto Silva, Eben North Broadbent, et al. Beyond trees: Mapping total aboveground biomass density in the Brazilian savanna using high-density UAV-lidar data. Forest Ecology and Management, 2021, 491: 119155. DOI:10.1016/j.foreco.2021.119155 |
44. | S. Magnussen, C. Kleinn, L. Fehrmann. Wood volume errors from measured and predicted heights. European Journal of Forest Research, 2020, 139(2): 169. DOI:10.1007/s10342-020-01257-9 |
45. | Jessica Esteban, Ronald E. McRoberts, Alfredo Fernández-Landa, et al. A Model-Based Volume Estimator that Accounts for Both Land Cover Misclassification and Model Prediction Uncertainty. Remote Sensing, 2020, 12(20): 3360. DOI:10.3390/rs12203360 |
46. | Andrew T Hudak, Patrick A Fekety, Van R Kane, et al. A carbon monitoring system for mapping regional, annual aboveground biomass across the northwestern USA. Environmental Research Letters, 2020, 15(9): 095003. DOI:10.1088/1748-9326/ab93f9 |
47. | Stefano Puliti, Jonathan P Dash, Michael S Watt, et al. A comparison of UAV laser scanning, photogrammetry and airborne laser scanning for precision inventory of small-forest properties. Forestry: An International Journal of Forest Research, 2020, 93(1): 150. DOI:10.1093/forestry/cpz057 |
48. | Marek Lisańczuk, Krzysztof Mitelsztedt, Karolina Parkitna, et al. Influence of sampling intensity on performance of two-phase forest inventory using airborne laser scanning. Forest Ecosystems, 2020, 7(1) DOI:10.1186/s40663-020-00277-6 |
49. | Maryam Poorazimy, Shaban Shataee, Ronald E. McRoberts, et al. Integrating airborne laser scanning data, space-borne radar data and digital aerial imagery to estimate aboveground carbon stock in Hyrcanian forests, Iran. Remote Sensing of Environment, 2020, 240: 111669. DOI:10.1016/j.rse.2020.111669 |
50. | Mathieu Fortin. Updating plots to improve the precision of small-area estimates: the example of the Lorraine region, France. Canadian Journal of Forest Research, 2020, 50(7): 648. DOI:10.1139/cjfr-2019-0405 |
51. | Andrew J. Lister, Hans Andersen, Tracey Frescino, et al. Use of Remote Sensing Data to Improve the Efficiency of National Forest Inventories: A Case Study from the United States National Forest Inventory. Forests, 2020, 11(12): 1364. DOI:10.3390/f11121364 |
52. | Annika Kangas, Terje Gobakken, Erik Næsset. Benefits of past inventory data as prior information for the current inventory. Forest Ecosystems, 2020, 7(1) DOI:10.1186/s40663-020-00231-6 |
53. | Ralph Dubayah, James Bryan Blair, Scott Goetz, et al. The Global Ecosystem Dynamics Investigation: High-resolution laser ranging of the Earth’s forests and topography. Science of Remote Sensing, 2020, 1: 100002. DOI:10.1016/j.srs.2020.100002 |
54. | R D Yanai, C Wayson, D Lee, et al. Improving uncertainty in forest carbon accounting for REDD+ mitigation efforts. Environmental Research Letters, 2020, 15(12): 124002. DOI:10.1088/1748-9326/abb96f |
55. | Steen Magnussen, Anne-Sophie Stelzer, Gerald Kändler. Population and Stand-Level Inference in Forest Inventory with Penalized Splines. Forest Science, 2020, 66(5): 537. DOI:10.1093/forsci/fxaa008 |
56. | Sean P. Healey, Zhiqiang Yang, Noel Gorelick, et al. Highly Local Model Calibration with a New GEDI LiDAR Asset on Google Earth Engine Reduces Landsat Forest Height Signal Saturation. Remote Sensing, 2020, 12(17): 2840. DOI:10.3390/rs12172840 |
57. | Ronald McRoberts, Erik Næsset, Christophe Sannier, et al. Remote Sensing Support for the Gain-Loss Approach for Greenhouse Gas Inventories. Remote Sensing, 2020, 12(11): 1891. DOI:10.3390/rs12111891 |
58. | Minna Räty, Mikko Kuronen, Mari Myllymäki, et al. Comparison of the local pivotal method and systematic sampling for national forest inventories. Forest Ecosystems, 2020, 7(1) DOI:10.1186/s40663-020-00266-9 |
59. | Kelly S. McConville, Gretchen G. Moisen, Tracey S. Frescino. A Tutorial on Model-Assisted Estimation with Application to Forest Inventory. Forests, 2020, 11(2): 244. DOI:10.3390/f11020244 |
60. | Erik Næsset, Terje Gobakken, Ronald E. McRoberts. A Model-Dependent Method for Monitoring Subtle Changes in Vegetation Height in the Boreal–Alpine Ecotone Using Bi-Temporal, Three Dimensional Point Data from Airborne Laser Scanning. Remote Sensing, 2019, 11(15): 1804. DOI:10.3390/rs11151804 |
61. | Ronald E. McRoberts, Erik Næsset, Sassan Saatchi, et al. Local validation of global biomass maps. International Journal of Applied Earth Observation and Geoinformation, 2019, 83: 101931. DOI:10.1016/j.jag.2019.101931 |
62. | Ronald E. McRoberts, Erik Næsset, Greg C. Liknes, et al. Using a Finer Resolution Biomass Map to Assess the Accuracy of a Regional, Map-Based Estimate of Forest Biomass. Surveys in Geophysics, 2019, 40(4): 1001. DOI:10.1007/s10712-019-09507-1 |
63. | Jessica Esteban, Ronald McRoberts, Alfredo Fernández-Landa, et al. Estimating Forest Volume and Biomass and Their Changes Using Random Forests and Remotely Sensed Data. Remote Sensing, 2019, 11(16): 1944. DOI:10.3390/rs11161944 |
64. | L C Melo, R Schneider, M Fortin. The effect of natural and anthropogenic disturbances on the uncertainty of large-area forest growth forecasts. Forestry: An International Journal of Forest Research, 2019, 92(3): 231. DOI:10.1093/forestry/cpz020 |
65. | Luca Birigazzi, Timothy G. Gregoire, Yelena Finegold, et al. Data quality reporting: Good practice for transparent estimates from forest and land cover surveys. Environmental Science & Policy, 2019, 96: 85. DOI:10.1016/j.envsci.2019.02.009 |
66. | José Antonio Navarro, Nur Algeet, Alfredo Fernández-Landa, et al. Integration of UAV, Sentinel-1, and Sentinel-2 Data for Mangrove Plantation Aboveground Biomass Monitoring in Senegal. Remote Sensing, 2019, 11(1): 77. DOI:10.3390/rs11010077 |
67. | Kangas, Räty, Korhonen, et al. Catering Information Needs from Global to Local Scales—Potential and Challenges with National Forest Inventories. Forests, 2019, 10(9): 800. DOI:10.3390/f10090800 |
68. | JD Kotze, HB Beukes, T Seifert. Essential environmental variables to include in a stratified sampling design for a national-level invasive alien tree survey. iForest - Biogeosciences and Forestry, 2019, 12(5): 418. DOI:10.3832/ifor2767-012 |
69. | Ana Aguirre, Miren del Río, Sonia Condés. Productivity Estimations for Monospecific and Mixed Pine Forests along the Iberian Peninsula Aridity Gradient. Forests, 2019, 10(5): 430. DOI:10.3390/f10050430 |
70. | Paul L Patterson, Sean P Healey, Göran Ståhl, et al. Statistical properties of hybrid estimators proposed for GEDI—NASA’s global ecosystem dynamics investigation. Environmental Research Letters, 2019, 14(6): 065007. DOI:10.1088/1748-9326/ab18df |
71. | Yingying Mei, Jingxiong Zhang, Wangle Zhang, et al. A Composite Method for Predicting Local Accuracies in Remotely Sensed Land-Cover Change Using Largely Non-Collocated Sample Data. Remote Sensing, 2019, 11(23): 2818. DOI:10.3390/rs11232818 |
72. | Svetlana Saarela, Sören Holm, Sean Healey, et al. Generalized Hierarchical Model-Based Estimation for Aboveground Biomass Assessment Using GEDI and Landsat Data. Remote Sensing, 2018, 10(11): 1832. DOI:10.3390/rs10111832 |
73. | Liviu T. Ene, Terje Gobakken, Hans-Erik Andersen, et al. Large-area hybrid estimation of aboveground biomass in interior Alaska using airborne laser scanning data. Remote Sensing of Environment, 2018, 204: 741. DOI:10.1016/j.rse.2017.09.027 |
74. | L.C. Melo, R. Schneider, M. Fortin. Estimating model- and sampling-related uncertainty in large-area growth predictions. Ecological Modelling, 2018, 390: 62. DOI:10.1016/j.ecolmodel.2018.10.011 |
75. | António Ferraz, Sassan Saatchi, Liang Xu, et al. Carbon storage potential in degraded forests of Kalimantan, Indonesia. Environmental Research Letters, 2018, 13(9): 095001. DOI:10.1088/1748-9326/aad782 |
76. | Ronald E. McRoberts, Erik Næsset, Terje Gobakken, et al. Assessing components of the model-based mean square error estimator for remote sensing assisted forest applications. Canadian Journal of Forest Research, 2018, 48(6): 642. DOI:10.1139/cjfr-2017-0396 |
77. | Stefano Puliti, Svetlana Saarela, Terje Gobakken, et al. Combining UAV and Sentinel-2 auxiliary data for forest growing stock volume estimation through hierarchical model-based inference. Remote Sensing of Environment, 2018, 204: 485. DOI:10.1016/j.rse.2017.10.007 |
78. | Mathieu Fortin, Rubén Manso, Robert Schneider. Parametric bootstrap estimators for hybrid inference in forest inventories. Forestry: An International Journal of Forest Research, 2018, 91(3): 354. DOI:10.1093/forestry/cpx048 |
79. | Steen Magnussen, Thomas Nord-Larsen, Torben Riis-Nielsen. Lidar supported estimators of wood volume and aboveground biomass from the Danish national forest inventory (2012–2016). Remote Sensing of Environment, 2018, 211: 146. DOI:10.1016/j.rse.2018.04.015 |
80. | Yasumasa Hirata, Naoyuki Furuya, Hideki Saito, et al. Object-Based Mapping of Aboveground Biomass in Tropical Forests Using LiDAR and Very-High-Spatial-Resolution Satellite Data. Remote Sensing, 2018, 10(3): 438. DOI:10.3390/rs10030438 |
81. | Stefano Puliti, Liviu Theodor Ene, Terje Gobakken, et al. Use of partial-coverage UAV data in sampling for large scale forest inventories. Remote Sensing of Environment, 2017, 194: 115. DOI:10.1016/j.rse.2017.03.019 |
82. | Sonia Condés, Ronald E. McRoberts. Updating national forest inventory estimates of growing stock volume using hybrid inference. Forest Ecology and Management, 2017, 400: 48. DOI:10.1016/j.foreco.2017.04.046 |
83. | He Yin, Asia Khamzina, Dirk Pflugmacher, et al. Forest cover mapping in post-Soviet Central Asia using multi-resolution remote sensing imagery. Scientific Reports, 2017, 7(1) DOI:10.1038/s41598-017-01582-x |
84. | Liang Xu, Sassan S. Saatchi, Aurélie Shapiro, et al. Spatial Distribution of Carbon Stored in Forests of the Democratic Republic of Congo. Scientific Reports, 2017, 7(1) DOI:10.1038/s41598-017-15050-z |
85. | Steen Magnussen, Fransisco Mauro, Johannes Breidenbach, et al. Area-level analysis of forest inventory variables. European Journal of Forest Research, 2017, 136(5-6): 839. DOI:10.1007/s10342-017-1074-z |
86. | Sören Holm, Ross Nelson, Göran Ståhl. Hybrid three-phase estimators for large-area forest inventory using ground plots, airborne lidar, and space lidar. Remote Sensing of Environment, 2017, 197: 85. DOI:10.1016/j.rse.2017.04.004 |
87. | Tuomo Kauranne, Anup Joshi, Basanta Gautam, et al. LiDAR-Assisted Multi-Source Program (LAMP) for Measuring Above Ground Biomass and Forest Carbon. Remote Sensing, 2017, 9(2): 154. DOI:10.3390/rs9020154 |
88. | Milutin Milenković, Sebastian Schnell, Johan Holmgren, et al. Influence of footprint size and geolocation error on the precision of forest biomass estimates from space-borne waveform LiDAR. Remote Sensing of Environment, 2017, 200: 74. DOI:10.1016/j.rse.2017.08.014 |
89. | Svetlana Saarela, Johannes Breidenbach, Pasi Raumonen, et al. Kriging prediction of stand-level forest information using mobile laser scanning data adjusted for nondetection. Canadian Journal of Forest Research, 2017, 47(9): 1257. DOI:10.1139/cjfr-2017-0019 |
90. | Victor Felix Strîmbu, Liviu Theodor Ene, Terje Gobakken, et al. Post-stratified change estimation for large-area forest biomass using repeated ALS strip sampling. Canadian Journal of Forest Research, 2017, 47(6): 839. DOI:10.1139/cjfr-2017-0031 |
91. | P. W. West. Simulation studies to examine bias and precision of some estimators that use auxiliary information in design-based sampling in forest inventory. New Zealand Journal of Forestry Science, 2017, 47(1) DOI:10.1186/s40490-017-0101-7 |
92. | Steen Magnussen, Jean-Pierre Renaud. Multidimensional scaling of first-return airborne laser echoes for prediction and model-assisted estimation of a distribution of tree stem diameters. Annals of Forest Science, 2016, 73(4): 1089. DOI:10.1007/s13595-016-0581-2 |
93. | Anna H. Ringvall, Göran Ståhl, Liviu T. Ene, et al. A poststratified ratio estimator for model-assisted biomass estimation in sample-based airborne laser scanning surveys. Canadian Journal of Forest Research, 2016, 46(11): 1386. DOI:10.1139/cjfr-2016-0158 |
94. | Svetlana Saarela, Sören Holm, Anton Grafström, et al. Hierarchical model-based inference for forest inventory utilizing three sources of information. Annals of Forest Science, 2016, 73(4): 895. DOI:10.1007/s13595-016-0590-1 |
95. | Ronald E. McRoberts, Qi Chen, Grant M. Domke, et al. Hybrid estimators for mean aboveground carbon per unit area. Forest Ecology and Management, 2016, 378: 44. DOI:10.1016/j.foreco.2016.07.007 |
96. | James Halperin, Valerie LeMay, Emmanuel Chidumayo, et al. Model-based estimation of above-ground biomass in the miombo ecoregion of Zambia. Forest Ecosystems, 2016, 3(1) DOI:10.1186/s40663-016-0077-4 |
97. | Mathieu Fortin, Rubén Manso, Rafael Calama. Hybrid estimation based on mixed-effects models in forest inventories. Canadian Journal of Forest Research, 2016, 46(11): 1310. DOI:10.1139/cjfr-2016-0298 |
98. | Qi Chen, Ronald E. McRoberts, Changwei Wang, et al. Forest aboveground biomass mapping and estimation across multiple spatial scales using model-based inference. Remote Sensing of Environment, 2016, 184: 350. DOI:10.1016/j.rse.2016.07.023 |
99. | Liviu Theodor Ene, Erik Næsset, Terje Gobakken, et al. Large-scale estimation of aboveground biomass in miombo woodlands using airborne laser scanning and national forest inventory data. Remote Sensing of Environment, 2016, 186: 626. DOI:10.1016/j.rse.2016.09.006 |
100. | Estela Inés Moyano. Theme in English and Spanish. English Text Construction, 2016, 9(1): 190. DOI:10.1075/etc.9.1.10moy |
101. | P. Beckschäfer, S. Schnell, C. Kleinn. Agroforestry. DOI:10.1007/978-981-10-7650-3_5 |